Machine Learning – COMPSTAT project
Machine learning is a research area at the frontier of informatics, statistics and domain science that has become ubiquitous in Physics since around 2015. ML techniques leverage the information from the data to learn complex models and perform data driven inference or reduce the data dimensionality to facilitate its exploration and the discovery of unforeseen correlations.At IN2P3, particularly we have a long history of working with heterogeneous and and ever growing datasets. This makes for perfect applications of ML algorithms to increase the speed of the simulations, perform high dimensional regressions or classifications, detect anomalies in the data, etc.
But the field of ML is also growing fast, making it hard to keep up with the latest developments for the big variety of applications in our labs. This is why the Machine Learning master projet at IN2P3 aims at building and enabling a ML community at IN2P3 to exchange on the latest developments and applications for
- cosmology
- particle and nuclear physics
- accelerator physics
Objectives
The main objectives of this projects are- Foster collaboration between IN2P3 labs and experiments on ML activities
- Share state-of-the-art knowledge between project members and spread it across IN2P3
- Encourage physicist to participate to ML conferences
- Document ongoing ML projects and HEP related ML tutorials
As such we organise a yearly workshop, encourage the publishing of the developed applications and contribute to sending researchers, engineers and students to ML conferences.
Most general information can be found on the project website : https://machine-learning.in2p3.fr.
National coordinators
- David Rousseau (IJCLab) – scientific lead
- Alexandre Boucaud (APC) – technical lead
APC activities
The ML activities at APC are coordinated by Prof. Yvonne Becherini and Alexandre Boucaud.
Applications are encompassing the diversity of physics supported by APC, with projects in particule physics on ATLAS, neutrino physics on KM3NeT and Dune, gamma-ray physics on HESS and CoMET/ALTO, gravitational-wave physics on LIGO/Virgo, CMB physics on QUBIC and cosmology on Euclid and Rubin/LSST.
Project website: https://machine-learning.in2p3.fr
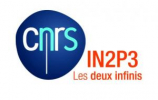